We’ve raised a total of $13M to accelerate AI’s ability to solve critical problems in the physical world! We are excited to partner with Carbide Ventures, Airbus Ventures, Peak XV (previously Sequoia Capital), Wavemaker Partners and Techstars on this mission.
Bifrost Overview Video
AI’s biggest frontier is the physical world
What we know as AI today—large language models, image generators, AI agents—is impressive, but it’s mostly confined to the digital realm. Yet the biggest and most pressing challenges aren’t on our screens; they’re in the physical world.
Think about industries that keep our world running - those that build, move and protect. They are facing significant challenges in labor shortages, supply chain breakages and geopolitical volatility. AI has only begun to scratch the surface of what it can truly achieve in these critical industries.
We’re here to bridge the gap and accelerate AI towards its biggest frontier—the physical world.
Now is the Time for Physical AI
Physical AI is where machines meet the real world. It’s about robots, sensors and systems that don’t just analyze data but impact real change to the physical environment.
Picture warehouse robots that manage logistics or defect detection systems that upkeep aging electrical grids. These aren’t just abstract algorithms running on servers but actual machines that interact with our physical world. Things don’t just get categorized or optimized but actually get done.
Robotics and perception systems aren’t new, but their current capabilities are still surprisingly limited. Why? Most rely on outdated, hard-coded rules that only work in narrow conditions. AI changes that. It breaks these limitations, opening up far greater potential—machines that are truly autonomous, adaptable, and able to tackle complex problems in diverse real-world scenarios.
The catch? You need data. A massive amount of it. And not just any data - the kind of domain-specific data physical AI requires is rare and incredibly hard to get.
The Data Bottleneck in Physical AI
While LLMs and diffusion models can leverage the vast amounts of text and image data available on the web, the challenge for physical AI is fundamentally different. You can’t scrape the internet to teach a robot the nuances of a car assembly line or how to spot critical defects on an electrical tower. This creates a massive bottleneck, with over 95% of enterprises struggling to build the high-quality training datasets they need.
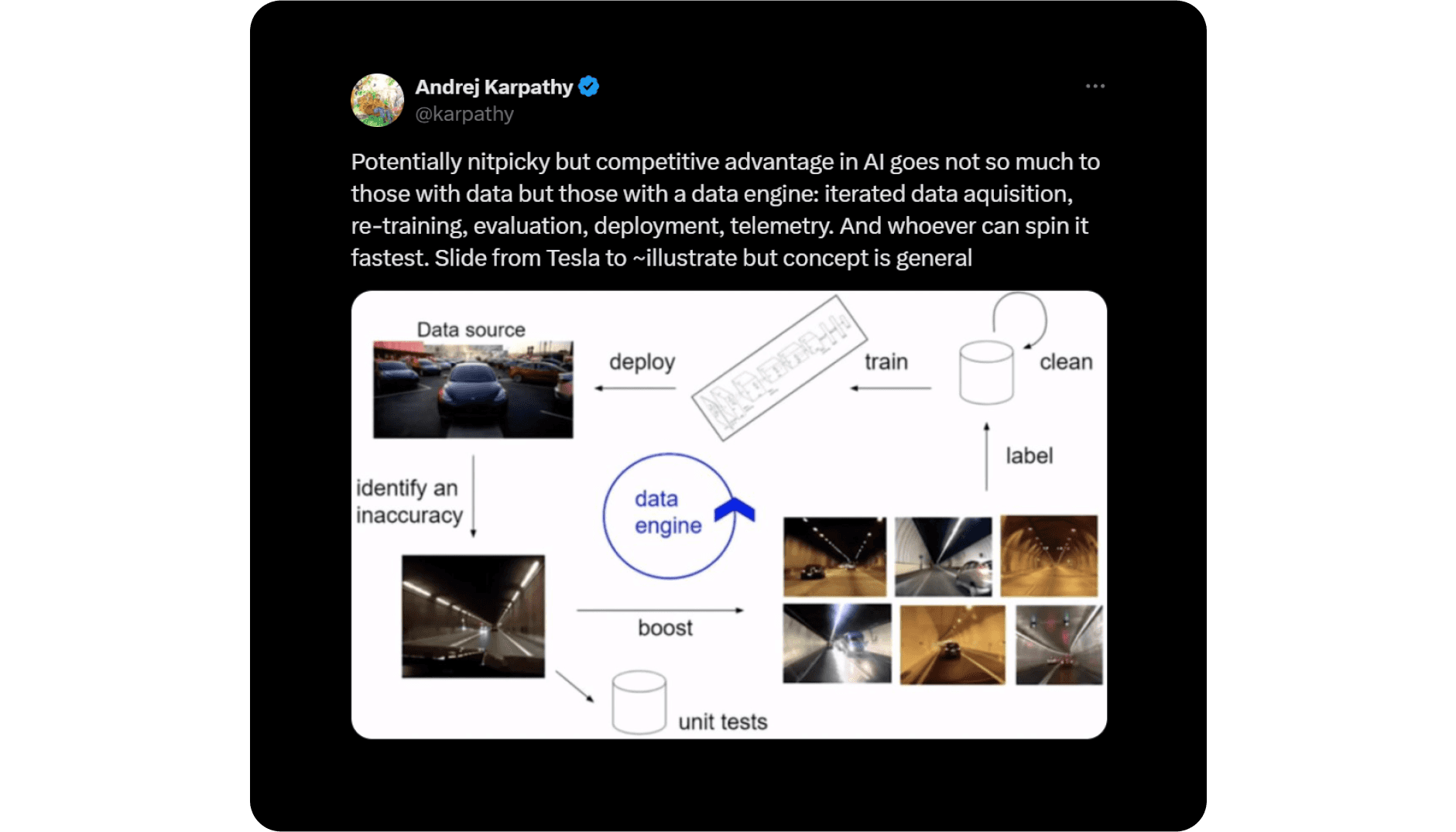
Andrej Karpathy's Tweet on Data Engines
Companies end up building costly, custom data engines—deploying fleets of robots across hundreds of locations, gathering millions of hours of footage, manually labeling data, and enforcing strict quality assurance to minimize human errors and bias.
This approach is brutal. It costs millions, takes years and is hard to scale. Often making it impossible for many teams to get started due to limited financial resources and time constraints.
Some teams can spin up smaller data engines, but they quickly hit a wall. Their models overfit to specific tasks and struggle to adapt to new scenarios. The problem circles back to real-world constraints like regulatory red tape, time pressure, and limited sensor fleets. The outcome is datasets that are too narrow, and lack the diversity needed for real-world generalization.
The Core Problem: AI Is Too Slow To Adapt
The real crux of the problem is that physical AI systems are too slow to adapt. The world is dynamic, and as soon as the environment or scenario changes, your AI becomes obsolete. Teaching a system to handle a new task or environment takes months of data collection and re-training.
Consider an autonomous boat: while it may navigate the Port of Los Angeles effectively, teaching it to operate in various global ports—each with distinct navigational markers and terrain features—can take months of additional deployments and data collection.
The result? AI systems get stuck in narrow use cases, never realizing their full economic or technological potential. We need a better way.
Bifrost: Enabling AI To Acquire Skills Rapidly & Adapt Effortlessly
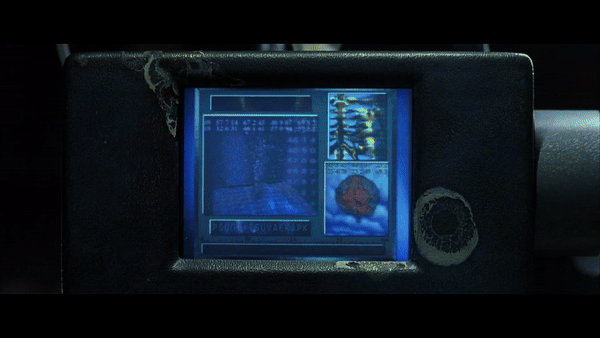
That iconic moment in the Matrix when Neo downloads Kung Fu directly into his mind.
What if AI could learn new skills as fast as Neo learns kung-fu in The Matrix?
At Bifrost, we’re creating the developer tools to make that possible—generative data engines that allow AI and robotics systems to rapidly acquire new abilities and adapt effortlessly. As François Chollet, creator of the Keras machine learning framework, puts it, real intelligence isn’t about mastering one task—it’s about how efficiently you can learn new ones.
Instead of spending months collecting real-world data, Bifrost lets teams generate it in minutes using simulated 3D worlds. We combine advanced 3D graphics with AI, so teams can build generative data engines that train, adapt, and test their systems faster than ever before.
Enabling robots to rapidly acquire specialized domain knowledge and skills for automotive assembly and inspection.
Traditional simulation tools either look fake or require expertise most teams don’t have. We fix both. Bifrost lets subject matter experts—who have no 3D modeling experience—build detailed, domain-specific scenarios. Need to adjust how a camera handles light on the ocean? Easy. Want to simulate object movement in a specific environment? Done. It’s precise where it needs to be, and simple where it should be.
The Bifrost platform simulating a autonomous patrol boat under different weather conditions
Speed, Affordability, and Reliability
What does this unlock?
For starters, teams can build AI faster. AI systems can rapidly adapt to new scenarios. What used to take months now takes days, and soon minutes. Teams can generate the exact data they need on demand, tackle a wider range of problems, unlock larger markets, and generate more revenue.
Second, we make physical AI cheaper. By minimizing the amount of upfront costly robotic platforms, extensive deployments, and manual data labeling, teams can reduce data collection and labeling costs by up to 70%. Fewer robots, fewer man-hours, less hassle.
Finally, Bifrost boosts reliability. Every physical AI system has limits, defined by its data and hardware. We help teams push those limits by testing in adversarial scenarios and spotting weaknesses before deployment. This proactive approach means identifying failure points early—long before they become real-world problems.
On A Mission To Accelerate Physical Intelligence
We have assembled a stellar team of AI scientists, robotics developers, computer graphics engineers, and Hollywood artists, to achieve a singular mission: to give AI teams the data and tools they need to solve complex problems in the physical world.
We've built AI that outperformed Google in clinical trials, developed autonomy for self-driving cars, won awards in computer graphics for photorealistic simulations, and rolled out nation-scale contact-tracing algorithms during the pandemic.
Over the past years we have been working with some of the most technically demanding organizations in the world that are solving some of the toughest problems. This comprises major U.S. government organizations and leading enterprises in heavy industry. One standout example is NASA JPL, with whom we have been collaborating on several initiatives, including data generation engines for Moon and Mars exploration.
Building physical AI is hard—there’s no getting around that. But it doesn’t have to be this hard. Bifrost is here to make the process faster, cheaper, and more reliable, so teams can focus on what matters. If that’s what you’re working on, let’s talk.
